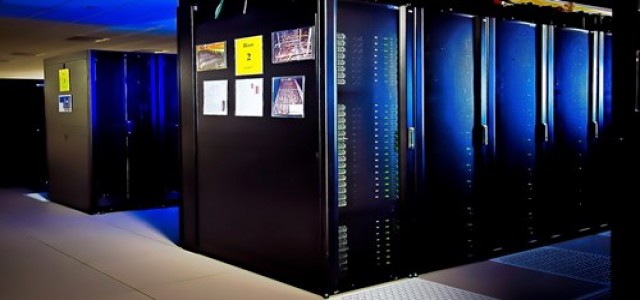
Intel has recently described its chip research related to transforming the computing experiences, with a focus on data that runs across the edge, core, and endpoints of systems.
According to Vivek De, Intel Labs’ Intel Fellow and Director of Circuit Technology Research, the new approach will serve as a means for Intel to shift its focus from hardware and programs to data and information. This requires a greater energy efficiency and high processing of devices where data has been generated, such as image sensors.
The main target of the research is to ensure an efficient computation technique, with a focus on several applications such as video analytics, machine vision, augmented reality, and robotics. It must overcome bandwidth, power, and memory limitations faced at various endpoints & other locations.
Few findings from the research can reportedly be adopted in the production process of new chips. The research paper presentation, which was covered in the Intel blog, was announced at the 2020 Symposia on VLSI Technology & Circuits.
11 researchers of the company, in a technical paper, have demonstrated the applications of all-digital BNN (Binary Neural Network) accelerator chip that is based on the 10-nm design of a fin field-effect transistor (FinFET) complementary metal-oxide-semiconductor (CMOS). Traditionally, in some power-constrained edge devices, BNNs have been analogs. However, BNN analogs have low accuracy level at making predictions and are less tolerant as compared to digital accelerators.
In the research paper, Intel delivers energy efficiency with the digital approaching as that of the analog in-memory. It also provides an enhanced scale for advanced processing. 617 TOPS (trillion operations per second) per watt have reached energy efficiency by using CNM (Compute Near Memory), inner product compute, & Near-Threshold Voltage operations.
One of the research papers included the local memory bandwidth doubling for AI, ML, and deep learning applications. The research paper also included a means to reduce the power that is required for video stream analysis based on deep learning, including how chips could be adopted with new algorithms to process visual inputs.
Source credit: